Student intern at Cox Collaboratory tests video analytics to improve bike parking at ASU

Imagine you’re an ASU student, heading off to class. You hop on your bike, knowing there’s a bike rack right outside the building. After furiously peddling your way there, you come to find that the rack is full.
Enter panic mode. Now you’re likely hot (it is Arizona, after all), on the verge of running late for class and left wondering where the next nearest rack is, dreading it will also be full.
After understanding these pain points from the ASU Parking and Transit Services team, the Cox Connected Environments Collaboratory set out to explore smart solutions to improve bike parking on campus.
Arizona State University's Tempe campus currently has more than 12,000 bicycle racks, none of which have any means to track utilization. The Cox Collaboratory team previously evaluated different sensor options for bike racks but found that installation, maintenance and ability to scale were all limiting factors. Their focus then turned to using video to overcome those limitations.
Rachel Hayden, a senior studying engineering mechanical systems at ASU and the collaboratory’s product and technology solutions analyst intern, spent time during her internship this summer creating a video analytics model using machine learning to be able to view and recognize bikes.
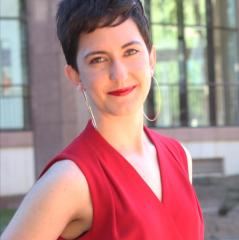
Step 1: Hayden had to choose between image classification and object detection. She selected object detection because “we’re looking more at detecting how many bikes there are rather than trying to distinctly tell the difference between a bike and some other object,” she said.
Step 2: Next, Hayden collected about 150 images of bicycles from search engines and uploaded them to Edge Impulse so the model knew what to analyze. “I collected images of singular bikes and multiple bikes that were grouped together,” Hayden said. “Along with a wide variety of backgrounds and different perspectives, looking at bikes from the front side, the back … and then getting different environments.”
Step 3. With each picture that Hayden uploaded, she used a labeling system to identify the bikes in each picture to better teach the model how to identify them. “I uploaded some pictures of scooters and some cars and just put no labels at all because it was just as important to be able to teach the model what was not a bicycle,” Hayden said.
Step 4. From there, Hayden was able to train the model using object detection from data that she had uploaded. With a final precision score of 60%, Hayden was ready to test.
Step 5. When the model was tested on data it had never seen before to see how well it would detect or count bikes in “real life,” the model received an accuracy score of 90.7%. To perform live classification, Edge Impulse allowed for use of a mobile phone for remote connection, which enabled Hayden to collect real-world images and test this new model around the ASU Tempe campus.
With such a high accuracy score, Hayden feels that the model’s success could potentially lead to monitoring overall utilization of racks by accurately counting how many bikes are currently there, optimizing rack placement around campus based on utilization data over time and offer more efficient bike parking by providing students insights into where spots are available.
“We’re proud of the incredible work that Rachel put into this project and her leadership in seeing it through from beginning to end,” said Brian Nickell, product and integration lead for smart communities at Cox2M. “We look forward to seeing how we can use this data and modeling to create a smarter and more efficient environment for ASU students, faculty and staff.”
The project offered win-win objectives for both ASU and the Cox Collaboratory, with some positives including:
For ASU:
- Envisioning how to better understand bike rack utilization to gauge use and availability.
- Learning how technology can assist in their efforts to encourage and promote more efficient bike transportation to reach sustainability goals.
For Cox Collaboratory:
- Prove video analytics solutions can and would work for this use-case.
- Evaluate machine learning platforms to streamline concepting and prototypes.
Learn more about other cutting-edge projects that Cox Collaboratory interns have done in the past.
More Science and technology

ASU professor wins NIH Director’s New Innovator Award for research linking gene function to brain structure
Life experiences alter us in many ways, including how we act and our mental and physical health. What we go through can even…

ASU postdoctoral researcher leads initiative to support graduate student mental health
Olivia Davis had firsthand experience with anxiety and OCD before she entered grad school. Then, during the pandemic and as a…
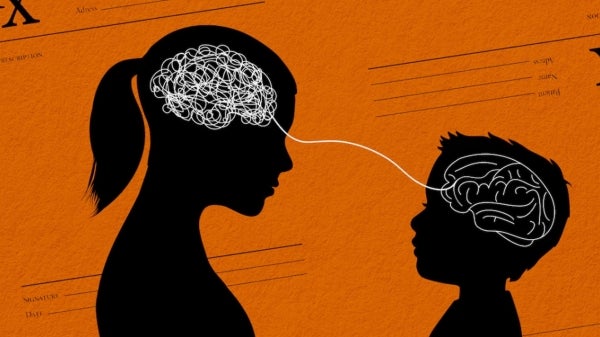
ASU graduate student researching interplay between family dynamics, ADHD
The symptoms of attention deficit hyperactivity disorder (ADHD) — which include daydreaming, making careless mistakes or taking…