Human brains teach AI new skills

ASU Regents Professor Ying-Cheng Lai is leading research efforts to improve machine learning strategies by using psychological principles about human memory to make artificial intelligence capable of dealing with more complex information to serve societal needs. Graphic created by Erika Gronek/ASU with photo by Deanna Dent/ASU
Artificial intelligence, or AI, is rapidly advancing, but it hasn’t yet outpaced human intelligence. Our brains’ capacity for adaptability and imagination has allowed us to overcome challenges and accomplish complex tasks for millennia, while AI is just getting started.
Arizona State University Regents Professor Ying-Cheng Lai thrives on working with complicated data and understanding chaos to advance human goals. His research focuses on how to make computing systems more capable of dealing with dynamic data, or information that changes over time.
“Memorizing sophisticated patterns is something we human beings can do — we recognize people’s faces and all kinds of things without much trouble. But if you ask a computer to do this, it will be very difficult,” says Lai, an electrical engineering faculty member in the School of Electrical, Computer and Energy Engineering, part of the Ira A. Fulton Schools of Engineering at ASU. “In the past 20 to 30 years, lots of progress has been made, but it’s still a little bit tricky, especially if the pattern is dynamic.”
Lai is taking inspiration from human memory to develop a dynamic system of machine learning memory using reservoir computing. The system can take in data, recognize and store patterns, and then project out those patterns over time.
This approach can help AI solve new problems previously seen as impossible with traditional static approaches. Dynamic machine learning memory could help us better use information from the past to predict the future in cases such as electric grid failures or critical climate change tipping points.
Along with his former electrical engineering doctoral student Ling-Wei Kong, Lai worked with Gene Brewer, a professor of psychology in The College of Liberal Arts and Sciences at ASU, to use biological memory strategies that work for humans to test new, sophisticated machine learning strategies.
The results of the work were published in the research journal Nature Communications.
Lai says giving an artificial neural network and AI algorithms human capabilities — "the special talents we have” — will help computing systems harness the best of human and artificial intelligence.
An interdisciplinary approach to solving complex problems
Most machine learning-based associative memories are designed for static patterns, such as pictures of cats. However, this approach cannot work with dynamic patterns that evolve continuously over time, just as a photo does not capture a cat’s life beyond that moment.
Lai wants to overcome the limitations of relying on static data for dynamic scenarios like predicting the future survival or extinction of a species. He says a basic requirement is that the machine learning architecture is capable of “self-evolution,” or automatically improving itself based on what it learns.
So Lai and Brewer looked at how the human brain handles this kind of task as inspiration, particularly how memories are stored and the recall strategies we use.
“We use biological insights to design our reservoir computer instead of coming up with mechanisms out of nowhere,” Lai says.
Brewer says psychological principles based on human thought processes and the distribution of memories in the brain can be useful to inform and improve machine learning algorithms.
“Equipped with this knowledge, a machine learning researcher who aims to emulate human cognition could develop methods for distributed representation within their network structures,” Brewer says.
Looking to the human brain for inspiration
Human cognition research has also often been conducted based on static information, such as remembering a specific word, Brewer says.
“What this type of prior research fails to account for is the dynamic nature of the world and our experiences in it,” Brewer says. “These experiences are much richer and also encoded into memory and can be remembered, similar to remembering a scene from a classic movie.”
While new research efforts focus on more natural dynamic situations people remember, Brewer says scientists have established that the human brain uses particular methods to record and retrieve memories. The main stages are encoding, maintenance and retrieval.
First, the brain establishes a memory in the encoding phase and then maintains or holds on to it when you’re not thinking about it.
When a person needs to remember something, or retrieve an encoded memory, it often depends on specific cues that help recreate the original experience from memory.
Many of the cues are associative, meaning they are based on connections between different things: faces and names, restaurants and cities, events and time, and other pairings.
“For example, if I associate a face with a name, then later see the person’s face (a cue), then it might help me remember their name (an associated piece of information),” Brewer says.
Artificial neural network systems like reservoir computing are designed to mimic the general concepts of biological memory and associative cues to work with dynamic data.
Testing dynamic machine memory
Lai and Kong presented the team’s reservoir computing system with hundreds of complicated, dynamic patterns. In the training phase, the system sorts the patterns into different sections called “basins” that coexist within one big “reservoir” that makes up the system memory.
The process is similar to what happens in your kitchen, which is comparable to the reservoir. A grocery trip yields ingredients for you to sort and organize on various shelves, or basins, in your kitchen’s refrigerator and pantry. Many ingredients are dynamic in that they have the potential to be transformed into all kinds of dishes.
“After training, this neural network is going to continuously produce time-varying information, or a dynamic evolution of the system,” Lai says.
In your kitchen, this would be similar to the ingredients making themselves into likely recipes for all the breakfasts, lunches, dinners, desserts and snacks you’ll have for the week.
Just as some of the brain’s inner workings are unknown, how a machine learning system sorts and processes information can be “mysterious,” Lai says.
“Understanding how machine learning works has been a daunting task,” Lai explains. “We can try to probe into the inner workings of this machine learning architecture and see what happens.”
To figure out how the system is storing the patterns, the team gave it various hints to try to recall the information. In addition to computing strategies like index cues, or using the name associated with a particular pattern, the system relies on associative memory strategies used by our brains.
Through various tests, the team found which hints were best at recalling a series of specific patterns. The researchers assessed trade-offs in speed and accuracy to discover viable strategies for dynamic pattern recall.
Next steps continue to advance machine learning
Lai’s research so far demonstrates the reservoir computing system can work with limited dynamic data, such as the few variables that drive the chaotic movement of a double pendulum.
Next, he hopes to better understand the basin structure that stores the patterns the reservoir computing system identifies in the data.
“We’re trying to understand the boundary structure right now to have a better understanding of how the memories start in this reservoir computing structure,” Lai says.
Further exploration into this topic could lead to more complex reservoir computing systems and more imaginative AI to help scientists and engineers solve dynamic societal challenges.
More Science and technology

Brilliant move: Mathematician’s latest gambit is new chess AI
Benjamin Franklin wrote a book about chess. Napoleon spent his post-Waterloo years in exile playing the game on St. Helena. John Wayne carried a set and played during downtime while filming “El…
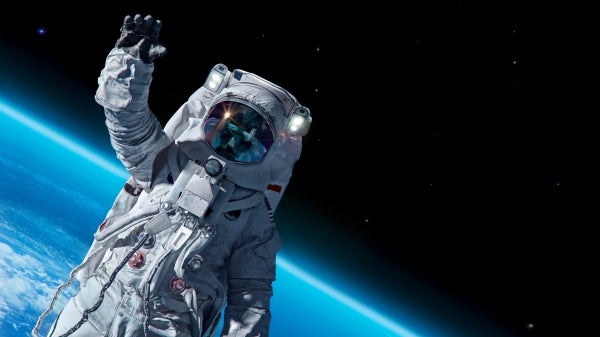
ASU team studying radiation-resistant stem cells that could protect astronauts in space
It’s 2038.A group of NASA astronauts headed for Mars on a six-month scientific mission carry with them personalized stem cell banks. The stem cells can be injected to help ward off the effects of…
Largest genetic chimpanzee study unveils how they’ve adapted to multiple habitats and disease
Chimpanzees are humans' closest living relatives, sharing about 98% of our DNA. Because of this, scientists can learn more about human evolution by studying how chimpanzees adapt to different…