CEOs of America, beware — artificial intelligence might have your number.
Analyzing CEO speech patterns, artificial intelligence now can detect when business leaders are lying or using deceptive language with 84% accuracy thanks to a data-driven machine-learning model, said a professor at Arizona State University’s W. P. Carey School of Business.
“It’s better and more accurate than a traditional lie detector,” said Jonathan Bundy, associate professor and Dean’s Council Distinguished Scholar for the W. P. Carey Department of Management and Entrepreneurship.
Bundy’s findings are part of a new study for which he partnered with four other academicsSteven J. Hyde, Eric Bachura, Richard T. Gretz and William Gerard Sanders were also co-authors on the research paper. , titled “The Tangled Webs We Weave: Examining the Effects of CEO Deception on Analyst Recommendations.”
They conclude that investors are financially impacted and organizations are overvalued when CEOs use deception. They also found that deception only works for CEOs up to a point, after which the continued use of deception can raise suspicion and doubt. They hope their study will raise accuracy levels in deception detection and lower susceptibility to deception.
ASU News spoke to Bundy about corporate governance, accountability and deceptive forms of communication.
Editor's note: Answers have been edited for length and clarity.
Jonathan Bundy
Question: Very interesting premise. Was there a case or scandal that prompted your research paper?
Answer: The project was born right after the Wells Fargo scandal in late 2016. We found it pretty incredible that financial markets and analysts were completely surprised by the scandal, despite numerous red flags discovered after the fact, both in the financial data being reported and in some of the language of the CEO and other leaders. We also found it interesting that after the scandal, analysts and markets reacted aggressively, downgrading the stock and putting pressure on the firm to fire the CEO and reset the culture.
We couldn’t help but wonder how helpful that pressure would have been before the scandal broke and how much damage was done simply because the people motivated to pay attention — e.g., stock market analysts — largely failed in their duties. Financial analysts get paid a lot of money to scrutinize firms to determine their “true value,” yet they completely missed signs of this massive scandal.
We wanted to know why. We wanted to see if we could use advanced machine learning to detect the possibility of deception perhaps better than analysts and other market observers.
Q: What type of research did you do to trace CEO deceptions?
A: There is extensive literature on deception from several different disciplines, including psychology, sociology, criminology, linguistics, philosophy and management. In terms of detecting deception, most of the research has been focused on physiological features — e.g., classic polygraph tests use blood pressure and skin conductivity changes to measure deception — or linguistic features, like with our study.
Research shows that deceivers typically use certain linguistic speech patterns, including using fewer singular pronouns, inconsistent verb tense and fewer words referring to sensory experiences — e.g., words that reflect motion, space or time. However, this research is only about 65% accurate in detecting deception, which is better than our baseline ability — typically, accuracy without any additional tools hovers around 47% — but still needs improvement. Using a machine-learning approach, we build on this prior research in linguistics and can detect deception with around 85% accuracy. We use 22 different linguistic features linked to deception to build our model. This is a significant improvement but also shows that there is room to grow.
We should also stress that “black box” issues arise due to the opaque decision-making processes of machine-learning models. These models prioritize prediction accuracy by randomly combining the 22 linguistic input features to maximize efficiency. As a result, it is hard to discern exactly why our model categorizes a specific text as deceptive or honest. This is just the nature of machine-learning models. You or I could read a text flagged as deceptive and likely not tell the difference from a non-deceptive text. The model considers all 22 features in combination, not individually, so the complexity of the prediction is well beyond anything we could perceive by just reading the document on our own.
Q: What usually drives a CEO to a pattern of deception?
A: To develop our measure of deception, we focused on fraudulent financial statements. These public accounting documents have been deemed by the SECU.S. Securities and Exchange Commission as purposefully false. Importantly, we do not focus on accounting mistakes or non-purposeful inaccuracies; we only look at actual fraud.
There has been significant research on why CEOs might commit fraud in their accounting statements. The answer is almost always the same: to improve stock market performance. This might be to hide something or to cover for mistakes or bad performance in the past, it might be to exaggerate good performance or to project positive performance into the future or it might be to make the firm look better compared to peers.
While we don’t investigate the antecedents of deception in the current study, the research is clear that deception is usually used to increase firm performance, and we build that assumption into our paper.
Q: Isn’t there an inherent danger in taking the human element out of this by allowing AI to make such a big decision?
A: Yes and no. Many of the dangers of AI in general, and in our case more specifically, are related to trust. Yes, our results show that we can closely detect deception. However, we cannot perfectly detect deception and likely never will be able to do so. So no one should completely trust any AI model or prediction. They are exactly that: a prediction, a likelihood, an inference. As such, we view our model as a tool to aid analysts, the media and other corporate observers to build a healthy skepticism and help them ask the right questions.
Additionally ... given the limitations of our understanding of the exact decision-making process in the machine-learning model, it is challenging to rule out potential biases or inaccuracies in specific contexts. For example, the model’s accuracy may diminish for certain demographics like minority groups, non-native English speakers or neurodivergent individuals. The model was trained using communications from a largely white and male population — U.S. corporate CEOs — so we would urge caution in thinking about its applicability to different audiences.
Interestingly, our training data also only includes those who were caught lying. So, it is possible that individuals who were not caught have fundamentally different linguistic patterns and that our model would be less accurate with these populations.
Q: Is there software now available on the market that companies can buy?
A: We have made the algorithm used in the study freely available on GitHub, and my co-authors also have a commercial version available.
Q: What’s next in terms of research on CEO deception?
A: There are a number of future research opportunities. As detailed above, much more is likely needed to understand the motivations or antecedents of deception. More is likely needed to understand how we might be able to avoid deception. And more is needed to understand individual differences between CEOs who deceive and those who do not. For example, our results provide correlational evidence that female and older CEOs engage in less deception. In contrast, correlational evidence suggests that higher-performing or award-winning CEOs tend to engage in more deception. We need to understand better why these differences might exist.
Using machine learning and AI to detect deception likely also means that machine learning and AI can be used to lie and deceive better. Now that our algorithm is available, it can probably be used to build new algorithms to hide deception. This is particularly true with more generative models and tools, like ChatGPT and others.
There was a similar problem with polygraph tests. Once people began to understand how polygraph tests worked, they could train their responses to beat the tests — e.g., work to keep their heart rate from changing while lying. Our model faces the same problem. Now that it is available, it can be used to beat itself. So research will have to continue iterating and improving on the model to keep it fresh and to adapt to continuous changes in artificial intelligence and machine learning.
Top photo courtesy iStock/Getty Images
More Science and technology

Brilliant move: Mathematician’s latest gambit is new chess AI
Benjamin Franklin wrote a book about chess. Napoleon spent his post-Waterloo years in exile playing the game on St. Helena. John Wayne carried a set and played during downtime while filming “El…
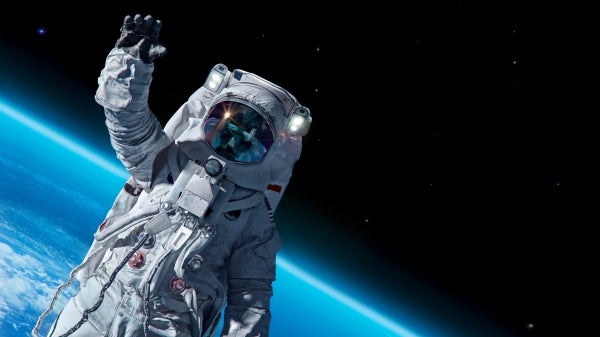
ASU team studying radiation-resistant stem cells that could protect astronauts in space
It’s 2038.A group of NASA astronauts headed for Mars on a six-month scientific mission carry with them personalized stem cell banks. The stem cells can be injected to help ward off the effects of…
Largest genetic chimpanzee study unveils how they’ve adapted to multiple habitats and disease
Chimpanzees are humans' closest living relatives, sharing about 98% of our DNA. Because of this, scientists can learn more about human evolution by studying how chimpanzees adapt to different…